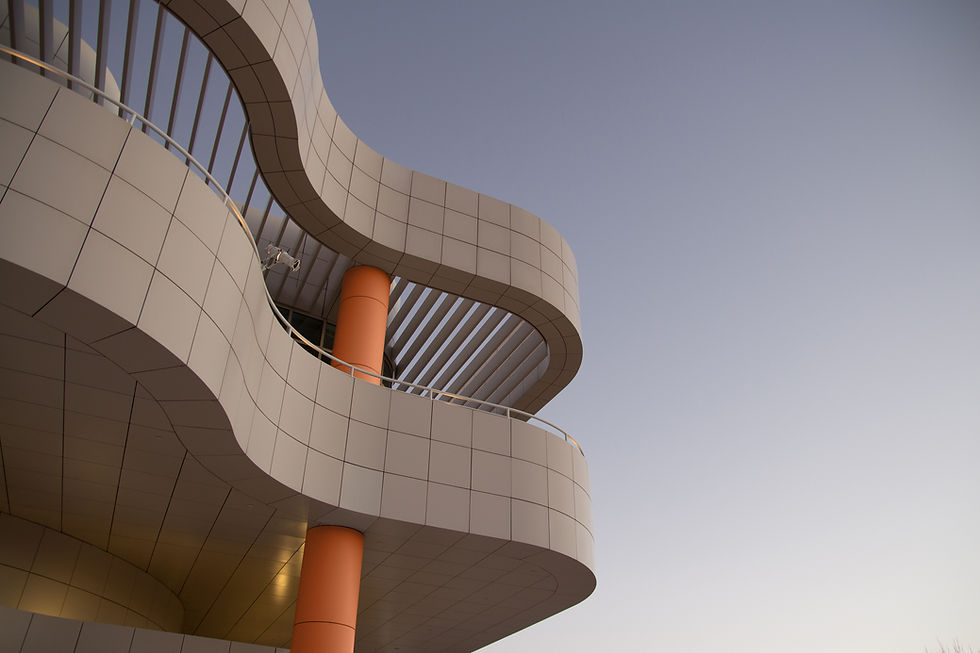
ASL Citizen: A New Dataset for Sign Language Technology
1
28
Imagine being able to look up an unfamiliar ASL sign by simply demonstrating it to your device. Whether you're a fluent signer or just beginning to learn, this type of interaction could transform accessibility in technology—think of ASL-compatible assistants like Siri or Alexa or search engines that understand signed queries. This vision is one step closer to reality thanks to the ASL Citizen dataset, a collaborative project we worked on with Microsoft Research, University of Washington, and University of Maryland. The authors of the study are Aashaka Desai, Lauren Berger, Fyodor O. Minakov, Vanessa Milan, Chinmay Singh, Kriston Pumphrey, Richard E. Ladner, Hal Daumé III, Alex X. Lu, Naomi Caselli, and Danielle Bragg.
The ASL Citizen project, published in NeurIPS 2023 Datasets and Benchmarks, provides the largest crowdsourced dataset of isolated ASL signs ever compiled. This innovative resource sets a new standard for machine learning research in sign language recognition, which is a cornerstone for creating ASL-integrated technology.
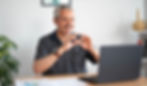
The Dataset: What Makes It Unique?
ASL Citizen contains over 83,000 videos of 2,731 ASL signs, recorded by 52 signers with diverse backgrounds and signing styles. Unlike many earlier datasets, which were often collected in controlled lab environments or scraped from online content, ASL Citizen videos were recorded by participants in real-world settings, reflecting the variability and richness of everyday signing.
Key features include:
Crowdsourced Contributions: Fluent ASL users recorded their own signing, with full consent and compensation.
Diversity: Signers come from 16 U.S. states, spanning different ages, genders, and ASL experience levels.
High Quality: Videos are carefully labeled and verified, ensuring accurate machine learning training data.
This dataset’s creation was guided by Deaf researchers and included culturally sensitive recruitment and consent practices.
Early tests with the ASL Citizen dataset showed that the system could accurately match a signed video to the correct entry in an ASL dictionary 63% of the time—nearly double the accuracy of earlier systems.
Paving the Way for ASL Technology
We focused our early testing of ASL Citizen on building technology for searching an ASL dictionary for a sign. Users can demonstrate a sign to a camera, and the system retrieves the closest matches from a digital dictionary. Early tests using the ASL Citizen dataset showed that the system could correctly identify the right sign on the first try 63% of the time—almost twice as accurate as earlier systems built with smaller or less diverse datasets.
But dictionary retrieval is only the beginning. This dataset could enable:
ASL-compatible voice assistants that respond to signed commands.
Search engines that understand ASL input.
Educational tools for ASL learners to practice signing interactively.
Strengthening Accessibility and Innovation
ASL Citizen exemplifies the power of collaboration between technology developers and the Deaf community. By prioritizing real-world needs and cultural awareness, we hope this work creates opportunities for signing communities to access technology in ways that feel intuitive and meaningful. With datasets like ASL Citizen, the gap between sign language users and digital technology can continue to close, paving the way for a more inclusive technological future.